Marinka Zitnik
Kempner Associate Faculty
Assistant Professor of Biomedical Informatics
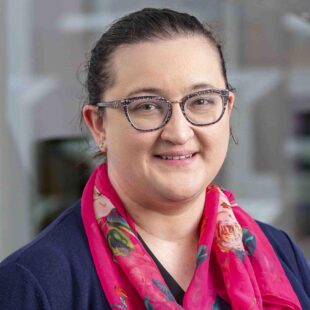
Contact Information
Subjects I Teach:
- Artificial Intelligence in Medicine
- Biomedical Informatics
Areas I Research:
About
Marinka Zitnik is an Assistant Professor in the Department of Biomedical Informatics at Harvard Medical School, Associate Faculty at the Kempner Institute for the Study of Natural and Artificial Intelligence, and Associate Member at the Broad Institute of MIT and Harvard University. Dr. Zitnik investigates machine learning with a current focus on learning systems informed by geometry, structure, and symmetry, and grounded in knowledge. This approach creates foundational models, including pre-trained, self-supervised, multi-purpose, and multi-modal models trained at scale to enable broad generalization. Dr. Zitnik has published extensively in top ML venues, such as NeurIPS, ICLR, and ICML, and leading scientific journals, including Nature, Nature Methods, and the Proceedings of the National Academy of Sciences (PNAS).
Her research received best paper and research awards from International Society for Computational Biology, International Conference on Machine Learning, Bayer Early Excellence in Science Award, Amazon Faculty Research Award, Google Faculty Research Scholar Award, Roche Alliance with Distinguished Scientists Award, Sanofi iDEA-iTECH Award, Rising Star Award in Electrical Engineering and Computer Science (EECS), and Next Generation Recognition in Biomedicine, being the only young scientist with such recognition in both EECS and Biomedicine. Dr. Zitnik was named Kavli Fellow 2023 by the National Academy of Sciences. Dr. Zitnik is an ELLIS Scholar in the European Laboratory for Learning and Intelligent Systems (ELLIS) Society. She is a member of the Science Working Group at NASA Space Biology. Dr. Zitnik co-founded Therapeutics Data Commons and is the faculty lead of the AI4Science initiative.
Research Focus
Dr. Zitnik’s research currently focuses on geometric deep learning, multimodal learning, knowledge graphs, foundation models, and biomedical AI. Her overarching goal is to lay the foundations for AI that contribute to the scientific understanding of medicine and therapeutic design, eventually enabling AI to learn on its own and acquire knowledge autonomously. Her group focuses on foundational innovation in machine learning with an emphasis on AI systems that are informed by geometry and structure and grounded in knowledge. This involves developing AI models, including pre-trained, self-supervised, and multi-modal models trained at scale to enable broad generalization.