With TxGNN, Kempner Researchers Introduce an AI “Dr. House” to Find Treatments for Rare Diseases
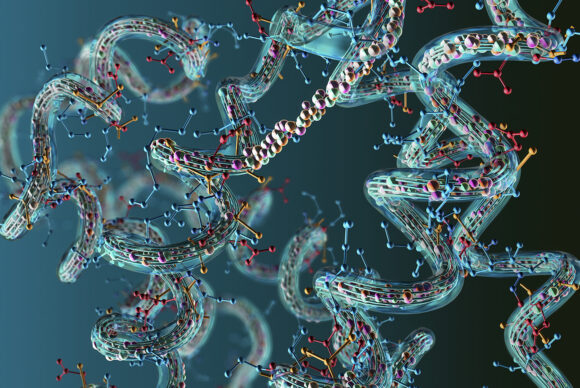
Kempner scientists are using powerful AI technology to identify potential drug-disease pairings that could help advance treatment for rare diseases.
In the medical drama “House,” which ran for eight seasons beginning in 2004, the titular Dr. House routinely solves mysteries involving rare diseases, drawing on a wealth of experience and reasoning skills to come up with unprecedented treatments. Now, researchers affiliated with the Kempner Institute at Harvard University have come up with an AI “Dr. House”: TxGNN, a graph foundation model that can predict if a drug might be able to help treat a rare disease, even if it has never before been tested on that disease.
This work, led by Marinka Zitnik, Kempner Institute associate faculty member and assistant professor of biomedical informatics at Harvard Medical School, has now been published in Nature Medicine.
“These diseases remain medical mysteries, and the lack of treatments is a major problem for those affected,” says Zitnik. “AI holds the potential to guide experimental studies in biological labs, helping to solve these mysteries.”
Harnessing AI to identify “explainable” drug-disease pairings
There are around 7,000 rare diseases known to medicine, and the overwhelming majority of them – 93 to 95 percent according to scientists – have no FDA-approved treatment whatsoever. Drug discovery is time-consuming and expensive, even for widespread diseases, so there is very little incentive for drug companies to study rare diseases that offer little potential to recoup investments.
To come up with possible treatments for such “orphan” diseases, doctors have traditionally employed a method like that of Dr. House: they mine their accumulated understanding of medicine and biology, often assisted by a chance discovery. A patient might happen to report an unexpected, beneficial side-effect of a drug they are taking for some other condition, leading to the discovery that the drug can be repurposed for a new application or treatment.
But relying on serendipity is slow and does little to actively address the needs of patients suffering from rare diseases. Even for more common diseases, finding new treatment options can help patients who don’t respond well to existing drugs or experience unwanted side effects.
To address this gap in treatment, Dr. Zitnik and her colleagues at Harvard Medical School and the Kempner Institute have developed TxGNN, an AI system that distills vast amounts of medical understanding into knowledge graphs, using these graphs to perform a “zero shot” prediction of the effectiveness of a drug for a rare disease.
Zero-shot learning means that TxGNN can predict the effectiveness of a drug-disease pairing even if it has never encountered this combination in the datasets it was trained on. According to Zitnik and her colleagues, TxGNN improves on the accuracy of its nearest AI competitors by 49.2% for indications and by 35.1% for contraindications.
Crucially, TxGNN is not a “black box” oracle: it is able to show its work, providing a rationale for each prediction. Predictive rationales extracted by TxGNN can help select the most promising drug candidates for downstream-focused biological experiments, enhancing the efficiency of experimental design. These rationales can also reveal gaps in the knowledge representation of the model, enabling researchers to identify missing or incomplete areas in the underlying datasets.
This kind of “explainable AI” — or “XAI” — is crucial for AI in medicine, and is also a major target of machine learning in general. It’s hard to trust a piece of software if we can’t understand the reasoning behind its recommendations. Moreover, a positive feedback loop between XAI and human experts is likely to boost research productivity, pointing researchers to possibilities that they might not have considered previously. In this way, XAI facilitates hypothesis generation and validation, bridging the gap between AI predictions and domain expertise in an actionable manner.
Learning from the web of medical knowledge
Zitnik and her colleagues employed cutting-edge machine learning techniques to distill medical and biological information into a “knowledge graph”: a data structure that represents the web of connections between drugs, DNA, cell signaling pathways, gene expression, medical records, clinicians’ notes, and other types of information relevant to diseases.
TxGNN was trained to perform a variety of prediction tasks using this trove of interconnected data. In one example, researchers asked the tool to identify patients that might respond well to a specific treatment or suggest therapies that would match their profile and specific conditions. In another test, researchers asked the tool to suggest pharmaceuticals that might interfere with the activity of proteins that participate in disease-related biochemical pathways.

To probe the model’s ability to emulate the suggestions of a human clinician, the researchers prompted TxGNN to find drugs for three rare conditions — a neurodevelopmental disorder, a connective-tissue disease, and a type of heart failure. The researchers then compared the model’s drug recommendations with current medical knowledge about how the suggested drugs work. In every example, the tool’s recommendations aligned with current medical knowledge.
Previously, only a human brain like that of the fictional Dr. House could hold such a large network of information together. And TxGNN likely exceeds the ability of any individual human in this realm. Human experts can often rely on extensive prior experience, but this experience can be biased or incomplete in ways that are hard to anticipate. With TxGNN, the knowledge base is known: it takes the form of the training data.
A path through the knowledge graph that humans can follow
Beyond the improvements in accuracy, TxGNN also represents a major advance for the field of XAI: it has the ability to explain why a specific intervention seems promising for a rare disease. Explainability is a major research area in modern AI and machine learning: state-of-the-art AI systems often provide users with accurate answers without revealing how they arrived at the answers.
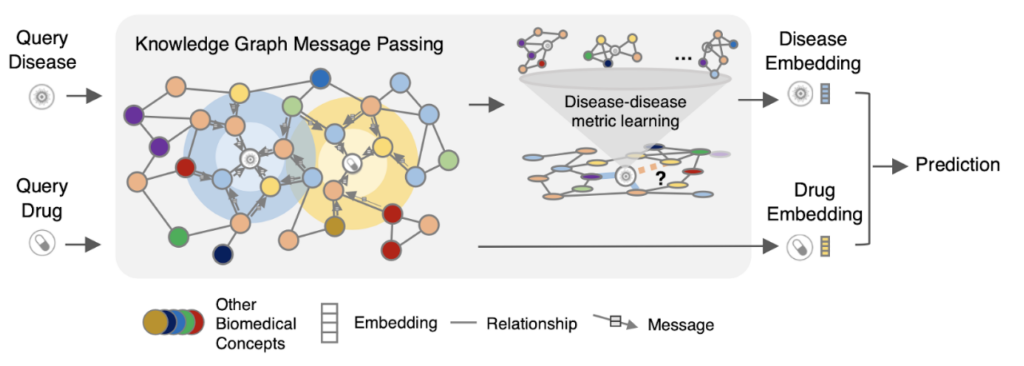
TxGNN explains its treatment recommendations by extracting paths between medical concepts in the knowledge graph that it was trained on. These sequences of associative “hops” between concepts can be understood by experts, providing a trail that human researchers and clinicians can follow to check for plausibility and safety.
Some AI systems do provide explanations, but they are not always user-friendly. Zitnik and colleagues dealt with this challenge by incorporating the latest research on user-centered XAI. Their freely available TxGNN Explorer tool is designed with clinician-researchers in mind.
Translational medicine supercharged by the Kempner cluster
Training and testing a model like TxGNN is highly computationally intensive. Zitnik and colleagues benchmarked the model across 17,000 tasks, each involving predicting potential drugs for a specific disease. The training began with pre-training the model on a medical knowledge graph using a graph neural network (GNN) architecture, which is optimized for processing relational, graph-structured datasets. This was followed by fine-tuning the model to predict which drugs might be effective for diseases, including those that currently lack approved treatments.
One of the most exciting features of TxGNN is its ability to perform zero-shot drug repurposing, predicting effective drugs for diseases it has not directly encountered during training by recognizing patterns from related diseases. This work and extensive benchmarking required an estimated compute of 2.69 x 10^20 FLOPs.
The computing resources brought together by the Kempner Institute offer unprecedented power to researchers at the forefront of both basic and translational research. Until very recently, only private tech companies had access to resources of this speed and scale.
“This research demands deep expertise in algorithm design, the ability to train complex models on vast datasets, and close collaboration between computer science and medicine,” says Zitnik. The Kempner’s compute resources and research community, she says, provide an essential resource to “drive innovation in biomedical AI and accelerate the pace of breakthroughs in disease treatment.”
With the publication of the new Nature Medicine paper by Zitnik and colleagues, we are already seeing the impact of such computing power on precision medicine. And, on the horizon are promising applications for treating real patients with information gleaned from this powerful AI “Dr. House”.
Read the full paper:
Huang, K., Chandak, P., Wang, Q., Havaldar, S., Vaid, A., Leskovec, J., Nadkarni, G.N., Glicksberg, B.S., Gehlenborg, N. and Zitnik, M., 2024. A foundation model for clinician-centered drug repurposing. Nature Medicine, pp.1-13.
This research was supported by National Science Foundation CAREER award (grant 2339524), National Institutes of Health (grant R01-HD108794), U.S. Department of Defense (grant FA8702-15-D-0001), Amazon Faculty Research, Google Research Scholar Program, AstraZeneca Research, Roche Alliance with Distinguished Scientists, Sanofi iDEA-TECH Award, Pfizer Research, Chan Zuckerberg Initiative, John and Virginia Kaneb Fellowship at HMS, Biswas Family Foundation Transformative Computational Biology Grant in partnership with the Milken Institute, HMS Dean’s Innovation Awards for the Use of Artificial Intelligence, Kempner Institute for the Study of Natural and Artificial Intelligence at Harvard University, and Dr. Susanne E. Churchill Summer Institute in Biomedical Informatics at HMS.