Kempner Institute Announces Recipients of 2024 Graduate Fellowships
Fifteen students awarded fellowships to pursue cutting-edge research at the intersection of natural and artificial intelligence
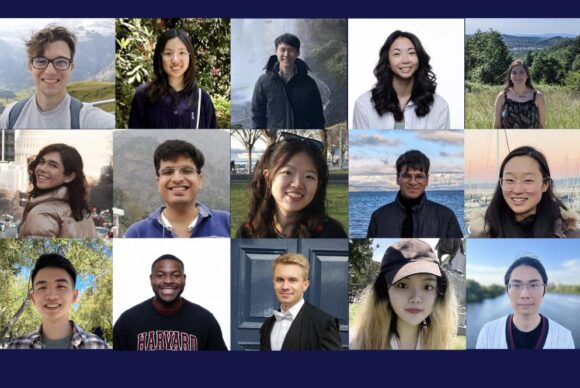
The Kempner's 2024 graduate fellowship recipients include eight incoming and seven continuing Harvard Ph.D. students representing six different disciplines, all pursuing research that advances intelligence in natural and artificial systems.
Cambridge, MA – The Kempner Institute for the Study of Natural and Artificial Intelligence today announced the names of 15 students chosen as the incoming 2024 cohort of Kempner Graduate Fellows.
The 2024 recipients of the Kempner graduate fellowship are: Rachit Bansal, George Cai, Lukas Fesser, Sara Fish, Kexin (Cindy) Luo, Alex Meterez, Depen Morwani, Daniel Yutaka Sprague, Chloé Huangyuan Su, Ikechukwu Uchendu, Valerie Wang, Catherine Yeh, Jason Yik, Yuyang Zhang, and Rosie Zhao.
“With the addition of this new cohort of graduate fellows, the Kempner will build upon its exceptional community of young scholars eager to advance the field of intelligence.”
Ella Batty, Assistant Director for Educational Programs
This year’s fellowship recipients include seven incoming and eight continuing graduate students enrolled across six Harvard graduate programs, and will bring the Kempner’s total roster of graduate fellows to 37.
“Graduate students come to the Kempner with their fresh perspectives to pursue some of the newest, biggest questions in intelligence research, and are essential to realizing the mission and vision of the institute,” said Professor Venkatesh Murthy, chair of the Kempner’s graduate fellowship selection committee.
While graduate fellows have a primary research focus aligned with the Kempner’s mission of understanding the basis of intelligence, the fellowship supports a diverse community of Ph.D. students, seeding new and innovative scientific discovery in departments and labs across the University. This year’s fellowship recipients will be enrolled in Harvard Ph.D. programs in the fields of Computer Science, Applied Math, Psychology, Neuroscience, Electrical Engineering and Biophysics.
Kempner graduate fellows receive mentorship, access to the Kempner’s computing resources and facilities, and funding up to and including the 4th year of graduate school.
Meet the Incoming 2024 Kempner Graduate Fellows
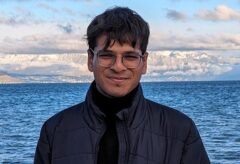
Rachit Bansal, Incoming Ph.D. Student, Computer Science
“Currently, large ML models are monolithic structures that are tedious to understand, improve, and serve. I am excited to design alternate paradigms that lead us towards modular and efficient models where adding features and fixes is easy.”
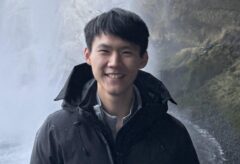
George Cai, Incoming Ph.D. Student, Neuroscience
“I want to understand how the brain builds internal models of the environment and generalizes knowledge across different contexts. I am excited for the opportunity to study the neural mechanisms of these abilities using modern deep networks and interpretability methods.”
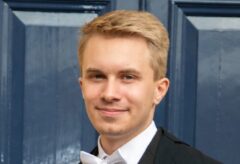
Lukas Fesser, Incoming Ph.D. Student, Applied Mathematics
“Geometric deep learning …allows us to address complex problems that were previously inaccessible with traditional deep learning techniques, for example in protein design or drug repurposing. My research [aims] to make these algorithms both more powerful and more reliable for human users.”
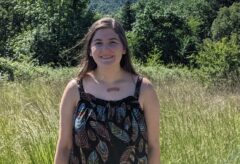
Sara Fish, Ph.D. Student, Applied Mathematics
“I find it interesting that general-purpose AI systems have become increasingly capable at decision-making and strategic reasoning tasks, and I aim to better understand their capabilities and limitations.”
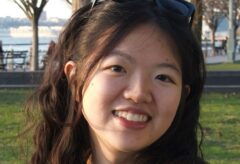
Kexin (Cindy) Luo, Incoming Ph.D. Student, Psychology
“My current research seeks to understand how systems can learn to process and integrate vision and language, specifically how neural networks combine these inputs to draw parallels with human multi-modal learning.”
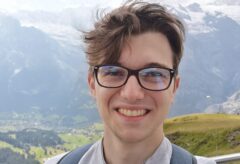
Alex Meterez, Incoming Ph.D. Student, Computer Science
“I am interested in establishing theoretical foundations for deep neural models, focusing on scale, efficiency and robustness. [I also] want to understand the current capabilities of large models, and how we can leverage these insights towards building neural networks that can reason in complicated scenarios.”
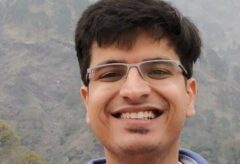
Depen Morwani, Ph.D. Student, Computer Science
“I am very excited about developing a better understanding of the optimization algorithms and their inductive biases in deep learning… [this] could not only lead to the development of more efficient algorithms, but also help in designing algorithms inherently biased towards safe, fair and robust features.”
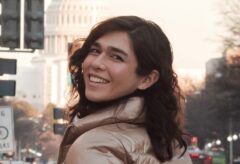
Daniel Yutaka Sprague, Incoming Ph.D. Student, Neuroscience
“Working at the intersection of neuroscience and modern machine learning allows me to take advantage of the cutting edge technologies of both fields to push the boundaries of how we understand the underlying mechanisms of neural computation.”
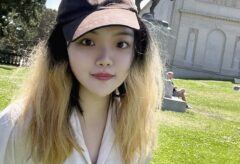
Chloé Huangyuan Su, Incoming Ph.D. Student, Computer Science
“My current research focus lies in reasoning and planning with foundation models, with applications in multi-robot or human-AI teams… What’s most exciting to me about my research and about the field of intelligence is how intelligence is efficiently, autonomously, and reliably extracted from data.”
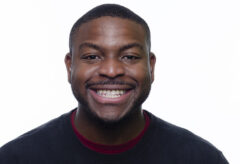
Ikechukwu Uchendu, Ph.D. Student, Computer Science
I am excited about how we can better use foundation models as agents in the real world that can improve over time with more experience. I’m particularly interested in exploring how these agents can automatically understand and interface with new tasks, while simultaneously tackling various sequential decision-making problems.”
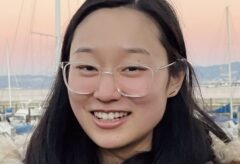
Valerie Wang, Incoming Ph.D. Student, Biophysics
I am excited to [use] methods from physics and mathematics to explore the neural mechanisms underlying biological computation. I am motivated by the idea that in furthering our comprehension of the brain and its operations, we can ultimately contribute to improved treatments for a spectrum of neurological disorders.”
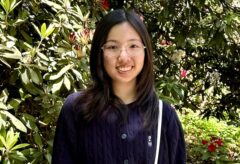
Catherine Yeh, Ph.D. Student, Computer Science
“I’m excited about designing visualization-based interpretability techniques and tools to recover phenomena inspired by natural intelligence in artificial networks. Guided by these explorations, I also hope to craft more productive, safe, and creative human-AI interactions.”
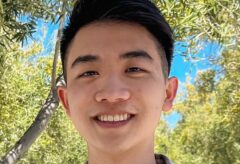
Jason Yik, Ph.D. Student, Computer Science
“Biology has already inspired successful AI methods such as convolution, reinforcement learning, and neural network models. By building algorithm + hardware systems that incorporate further biologically-inspired features such as sparsity and parallelism, artificial technology can approach the efficiency and performance of biological systems by looking more like biological systems.”
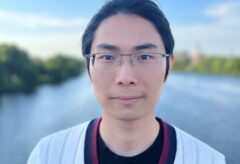
Yuyang Zhang, Ph.D. Student, Electrical Engineering
“The field of intelligence is particularly exciting to me because it offers the possibility to integrate natural intelligence with theory and engineering. More specifically, I am intrigued by the possibility to explain natural intelligence from theoretical and engineering perspectives and to enhance natural intelligence with theoretical and engineering insights.”
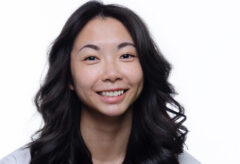
Rosie Zhao, Ph.D. Student, Computer Science
“A large motivation of my work is to make progress towards identifying general engineering principles for eliciting desirable properties like good performance or robustness from current [ML] models. Inventing new algorithms is always exciting, but taking the time to focus on understanding the algorithms we already have can often yield practical gains and fuel insights for further technological development.”
About the Kempner
The Kempner Institute seeks to understand the basis of intelligence in natural and artificial systems by recruiting and training future generations of researchers to study intelligence from biological, cognitive, engineering, and computational perspectives. Its bold premise is that the fields of natural and artificial intelligence are intimately interconnected; the next generation of artificial intelligence (AI) will require the same principles that our brains use for fast, flexible natural reasoning, and understanding how our brains compute and reason can be elucidated by theories developed for AI. Join the Kempner mailing list to learn more, and to receive updates and news.