Rethinking Generative Models in the Age of Science
Joey Bose
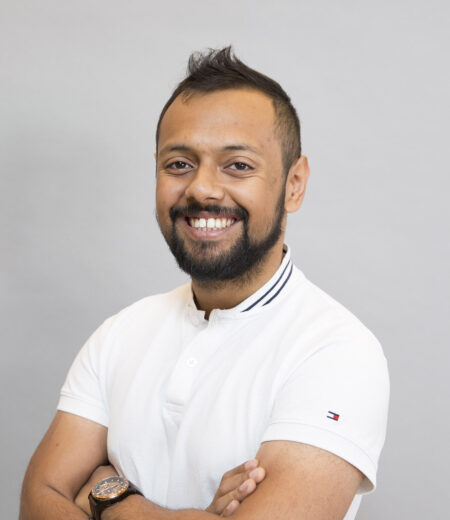
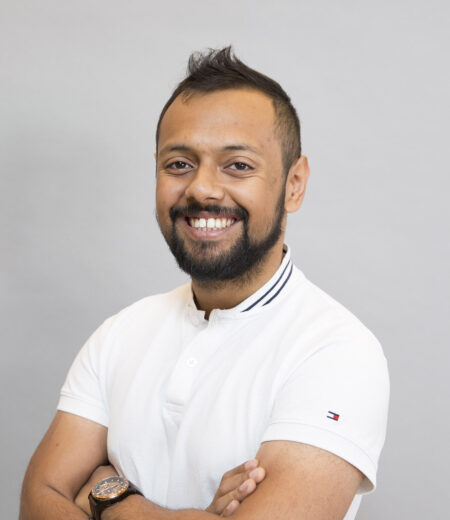
Abstract: Our natural world is governed by seemingly simple laws that often give rise to a rich tapestry of phenomena, such as complex physical, chemical, and biological processes that produce highly structured data. A better understanding of such scientific processes to the extent that they can be simulated at scale using machine learning has the potential to deliver revolutionary impact across several fields—already inaugurated by recent Nobel Prizes in Physics and Chemistry. This raises the exciting possibility of scientific applications experiencing their own GPT-4 GenAI moments, and leading to scientific breakthroughs that create billions of dollars in value through the creation of entirely new business markets like computational drug and material design. However, unlike data-rich domains such as images, delivering on the currently unfulfilled promise of scientific discovery on real problems requires the development of a new generation of probabilistic and generative modeling methods. In this talk, I outline the key challenges inherent to scientific domains that invite new approaches to build generative models to be robust generative samplers for amortized sampling of Boltzmann densities. This sets the stage to create a blueprint for building the next wave of generative models for scientific discovery, by shedding light on how current approaches explicitly encode and exploit scientific domain knowledge to successfully elevate models to their appropriate geometric spaces thereby unlocking the full performance arsenal of modern generative models.
Speaker Bio: Joey Bose is a Post-Doctoral Fellow at the University of Oxford working with Michael Bronstein and an Affiliate member of Mila. He completed his PhD at McGill/Mila under the supervision of Will Hamilton, Gauthier Gidel, and Prakash Panagaden. His research interests span AI4Science, Generative Modelling, and Differential Geometry for Machine Learning with a current emphasis on the theory and practice of building geometric generative models for scientific applications. Previously, he completed his Bachelor’s and Master’s degrees from the University of Toronto. His work has been featured in Forbes, New York Times, CBC, VentureBeat, and other media outlets and was generously supported by the IVADO PhD and the NSERC Post-doc Fellowships.