Towards Principled Post-Training of Large Language Models
Speaker: Banghua Zhu
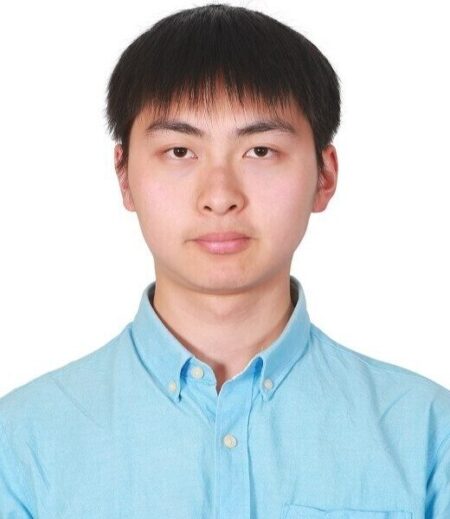
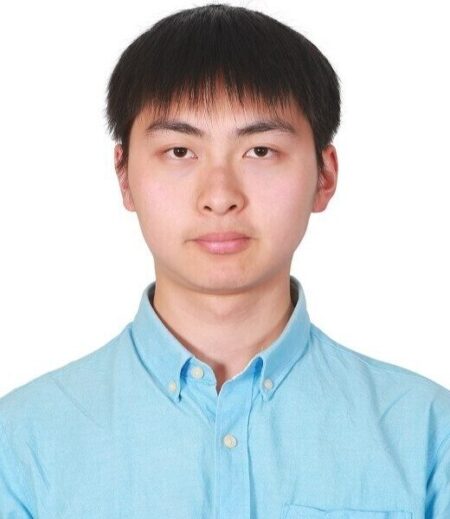
Abstract
Reinforcement Learning from Human Feedback (RLHF) is a pivotal technique that aligns large language models (LLMs) closely with human-centric values, and has created several leading LLMs, including GPT-4, Claude and Llama 2. The first step of RLHF involves learning human values using a reward model from ranking data. It is observed that the performance of the reward model degrades after one epoch of training, and optimizing the language model too much against the learned proxy reward model hinders the true objective. This talk delves into these issues, leveraging the theoretical insights from statistical decision theory to design improved reward learning algorithms. We also introduce advanced prompting techniques that generate high-quality open-source dataset for RLHF. By combining the high-quality RLHF dataset with our improved RLHF algorithms, we created the open-source language model Starling-7B, which ranks first among all 7B models according to human evaluation in Chatbot Arena.
11:10 am to 12:10 pm | Science and Engineering Complex (SEC), SEC 1.413
SPEAKER BIO
Banghua Zhu is a final-year PhD student at Berkeley, advised by Professor Michael I. Jordan and Jiantao Jiao. Banghua’s research focuses on statistics and information theory, with applications in contract theory, noisy computing, robust statistics, reinforcement learning, large language models and machine learning systems. He is a recipient of the David Sakrison Memorial Prize for outstanding PhD research at Berkeley EECS.