In Two Nature Publications, Kempner’s Marinka Zitnik Offers New Frameworks for Using AI to Advance Scientific Discovery
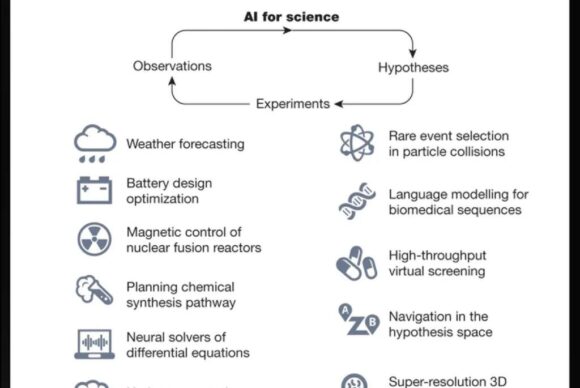
Artificial Intelligence is poised to reshape scientific discovery by augmenting and accelerating research at each stage of the scientific process. (“Scientific discovery in the age of artificial intelligence” originally published in Nature, is licensed under CC 4.0.)
From predicting protein folding to designing novel drugs, and even controlling nuclear reactors, artificial intelligence (AI) is being integrated into high-stakes research questions across the natural sciences. Still, we’ve barely scratched the surface of AI’s potential in these fields, according to Kempner Institute associate faculty member Marinka Zitnik.
In two recent works published in Nature and Nature Machine Intelligence, Zitnik and her collaborators offer new frameworks for making sense of today’s vast AI landscape, as well as for applying and adapting existing AI tools to aid in the scientific process, and helping to solve complex problems in the natural sciences.
Understanding the role of AI in scientific discovery
In “Scientific discovery in the age of artificial intelligence,” a review published in Nature, Zitnik led a team of researchers across 38 institutions and three continents to highlight how machine learning breakthroughs from the past decade can help scientists throughout the stages of the scientific process. For example, by leveraging AI’s strengths at handling information at scale to distill insights from millions of past publications and search vast sets of possible hypotheses, scientists are able to identify shared principles across scientific fields and generate novel hypotheses.
“The scientific method has been around since the 17th century, but the techniques used to generate hypotheses, gather data, perform experiments, and collect measurements can now all be enhanced and accelerated through the thoughtful and responsible use of AI,” Zitnik said in a recent interview with the Harvard Medical School, where she is an assistant professor of biomedical informatics.
Still, substantial challenges remain to harnessing AI’s full potential in these domains. Handling diverse multimodal data from images and structures to sequences, and fully understanding how powerful but opaque “black-box” models work, will require significant technical innovation. Zitnik advocates for cross-disciplinary collaborations that integrate software and hardware engineers with scientific research teams, as well as leveraging partnerships between industry and academia. “Addressing the challenges will require new modes of thinking and collaboration,” Zitnik and her collaborators write. “Moving forward, we have to change how research teams are formed.”
A deeper look into pre-training paradigms
In “Structure-inducing pre-training,” another recent article in Nature Machine Intelligence, Zitnik and her team conduct a comprehensive review of algorithmic innovations known as pre-training methods. Pre-trained models, especially large language models such as GPT-4, are transforming deep learning. Instead of training many task-specific models, we can now adapt a single pre-trained model to many tasks via fine-tuning. Pre-training teaches a model to identify patterns or make predictions based on an initial task or set of data; this pre-trained model can then be fine-tuned for use on subsequent tasks or data sets.
While pre-training has been an important innovation in training models, there remains considerable uncertainty as to exactly why pre-training helps improve performance for fine-tuning tasks. This is especially true when attempting to adapt language-model pre-training to domains outside of natural language, like the kind of multimodal data used in the natural sciences.
In their paper, Zitnik and her collaborators reveal gaps in our understanding of how pre-training methods structure the “representation space”— the set of features that most accurately represents the data— learned by the model. They then offer a new framework for pre-training, which builds in explicit user guidance to direct the structure of the knowledge learned by the pre-trained model.