Announcing 2024 Kempner Institute Research Fellows
Six innovative, early-career scientists awarded fellowships to work on projects that advance the fundamental understanding of intelligence
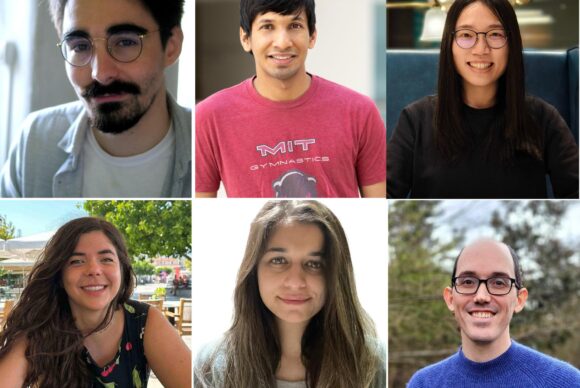
The 2024 Kempner Research Fellowship recipients are (top row, from left) Thomas Fel, Mikail Khona, Bingbin Liu, and (bottom row, from left) Isabel Papadimitriou, Noor Sajid, and Aaron Walsman.
Cambridge, MA – The Kempner Institute for the Study of Natural and Artificial Intelligence at Harvard is pleased to announce the recipients of its 2024 Kempner Institute Research Fellowships. The 2024 recipients are Thomas Fel, Mikail Khona, Bingbin Liu, Isabel Papadimitriou, Noor Sajid, and Aaron Walsman.
All six fellowship recipients are early-career scientists, representing a diversity of backgrounds and expertise, who are working on novel research questions at the intersections of natural and artificial intelligence.
Each fellow will serve for a term of up to three years and will receive salary and research funds, office space, and mentorship. Fellows set their research agenda but are strongly encouraged to work between fields and to collaborate with experts at the Kempner Institute and throughout Harvard University.
2024 Kempner Research Fellows
Thomas Fel researches large vision models, particularly their explainability and how they align with human vision. Motivated to uncover the secrets behind the exceptional ability of vision models to generalize, Fel blends computational techniques with insights from neuroscience to better grasp the inner workings of these models. This interdisciplinary approach not only aims to enrich the understanding of artificial intelligence but also positions this knowledge as a tool for probing into human intelligence.
Mikail Khona aims to develop tools and approaches to interpret and improve large foundational models. He uses an empirical approach, studying phenomena in these models and reverse-engineering them to identify their origins, attempting to trace back these behaviors to specific aspects of the model’s architecture, training data, or learning algorithms. This approach can improve the robustness and efficiency of these models and inform future model design. In addition, Khona’s work explores the intersection of biology and AI and examines the extent to which deep neural networks provide a natural language to describe biological phenomena.
Bingbin Liu is interested in the mathematical and empirical science of machine learning phenomena. Her research uses simple abstractions as testbeds for understanding complex systems, typically beginning with theoretical analyses and subsequently applying these insights to practical scenarios. Her work has focused on understanding the design choices in self-supervised learning, as well as exploring the capabilities and limitations of Transformers in reasoning. Looking forward, she is especially interested in investigating the factors affecting training efficiency and the reliability of models at inference time.
Isabel Papadimitriou works on understanding and defining the capabilities of large language models in relation to the human language system. Her work centers on two questions, bringing together the human and artificial faculties for language. Firstly, how do large language models work? To this end, she uses analyses from language science and applies them to artificial language learners in order to analyze the language system that LLMs latently encode. Secondly, how can a successful artificial language learner inform our knowledge of the human language system? She empirically tests the limits of artificial language learning under different conditions, to expand our hypothesis space about how human language learning and representation might function.
Noor Sajid aims to imbue artificial agents with the adaptability seen in biological intelligence, enabling these systems to apply learned knowledge to a variety of tasks within small-scale training data regimes. Accordingly, her research is grounded in developing generative models for understanding and mimicking biological decision-making to investigate how artificial agents can adapt to and learn from environmental perturbations, similar to humans and animals. Going forward, she plans to expand these models and delve deeper into the dynamics that facilitate the flexible utilization of acquired information.
Aaron Walsman investigates online information gathering and memory for agents in interactive environments. This work is primarily concerned with complex partially observable settings where an agent must reason about the limits of the information available to them and decide how to conduct further exploration to find the new information necessary to solve a particular task. The long-term goal of this research is to develop AI systems that intelligently reason over very long time horizons by selectively retaining the most important information from the past in order to make plans for improving their understanding in the future.
About the Kempner
The Kempner Institute seeks to understand the basis of intelligence in natural and artificial systems by recruiting and training future generations of researchers to study intelligence from biological, cognitive, engineering, and computational perspectives. Its bold premise is that the fields of natural and artificial intelligence are intimately interconnected; the next generation of artificial intelligence (AI) will require the same principles that our brains use for fast, flexible natural reasoning, and understanding how our brains compute and reason can be elucidated by theories developed for AI. Join the Kempner mailing list to learn more, and to receive updates and news.
PRESS CONTACT:
Deborah Apsel Lang | (617) 495-7993 | kempnercommunications@harvard.edu