Announcing 2023 Kempner Institute Research Fellows
Eight innovative, early-career scientists awarded fellowships to work on projects that advance the fundamental understanding of intelligence
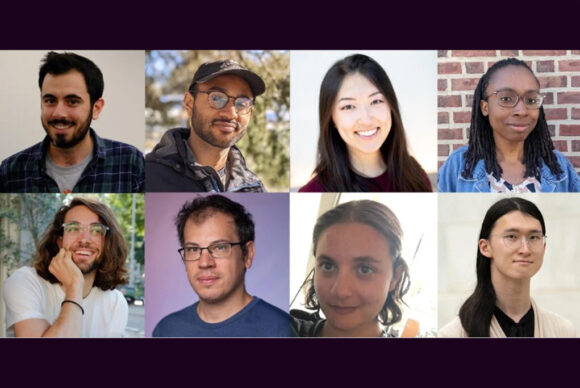
The 2023 Kempner Institute Research Fellowship recipients are (clockwise from left) David Brandfonbrener, Wilka Carvalho, Jennifer Hu, Ilenna Jones, Binxu Wang, Naomi Saphra, Eran Malach, and T. Anderson Keller.
Cambridge, MA – The Kempner Institute for the Study of Natural and Artificial Intelligence at Harvard is pleased to announce the recipients of its inaugural Kempner Institute Research Fellowships. The 2023 recipients are David Brandfonbrener, Wilka Carvalho, Jennifer Hu, Ilenna Jones, T. Anderson Keller, Eran Malach, Naomi Saphra, and Binxu Wang.
All eight fellowship recipients are early-career scientists, representing a diversity of backgrounds and expertise, who are working on novel research questions at the intersections of natural and artificial intelligence.
Each fellow will serve for a term of up to three years and will receive salary and research funds, office space, and mentorship. Fellows independently set their research agenda but are strongly encouraged to work between fields and to collaborate with experts at the Kempner Institute and throughout Harvard University.
David Brandfonbrener studies scalable approaches to solving problems in control as well as decision-making based on deep learning. His research generally starts from empirical observations of deep learning systems and from there derives theories and experimental confirmation to come to a more complete understanding of empirical phenomena. In keeping with the Kempner Institute’s mission, this work seeks to understand how and when intelligent behavior can arise from large-scale algorithmic learning.
Wilka Carvalho aims to develop theories for human learning and generalization based on evidence that humans leverage predictive knowledge about objects and agents to rapidly adapt to new situations. His research explores the computational benefits of developing AI agents that learn to make predictions over discovered object representations. He hopes to build on this work to develop novel algorithms that leverage “worlds models” and “successor features” to better discover and exploit representations of both objects and agents. This has the potential to both improve AI’s ability to rapidly generalize, and to inspire novel computational accounts for how brains learn, compose, and transfer predictive knowledge and behavior.
Jennifer Hu aims to understand how language works in the mind and brain. How do humans communicate so flexibly and effectively, and how does this happen given limited cognitive resources? Jennifer’s work approaches these questions using computational modeling, machine learning, and human experiments, serving the dual goals of reverse-engineering the human mind and safely advancing artificial intelligence.
Ilenna Jones uses detailed neuron models to study how dendritic properties contribute to a neuron’s computational capability as well as the neuron’s ability to maximize this capability through biologically plausible learning rules. Her work grounds theoretical models in biological detail, elucidating what neurons computationally permit at the circuit, network, and population levels. Through this research, Ilenna aims to use the implementable details of neurons to shape a normative understanding of neuronal capability, thus expanding our understanding of the brain’s computational capacity and ability to learn.
T. Anderson Keller undertakes research that focuses on understanding the abstract inductive biases which make natural intelligence efficient and generalizable — thereby allowing for the development of artificial intelligence with the same beneficial properties. To accomplish this, his work develops novel artificial neural network architectures which exhibit natural neural representational structure (such as topographic organization or synchronous spatiotemporal dynamics), and subsequently evaluates the computational implications of this structure. Through this process, his research aims to increase our understanding of the computational role of observed natural structure, while simultaneously making modern artificial intelligence behave more naturally.
Eran Malach undertakes research focused on developing a mathematical theory of deep learning. He studies the learning capabilities of neural networks, with an emphasis on exploring what makes neural networks succeed or fail in solving complex tasks. By investigating the effectiveness of different learning methods and exploring the limitations of deep learning models, his work aims to better understand the strengths and weaknesses of neural networks. This research promises to provide insights into how simple learning rules can lead to complex intelligent behavior.
Naomi Saphra studies the development of interpretable structures over the course of training in natural language processing (NLP) models, in order to gain a deeper understanding of emergent behavior in neural networks. By elucidating how large neural networks learn and behave, as well as how and why they fail, her research aims to make modern machine learning systems both interpretable and controllable. At the Kempner Institute, she will collaborate with researchers who work with other data modalities or in learning theory, to unify her linguistically-motivated empirical approach with other frameworks.
Binxu Wang works at the intersection of artificial intelligence and visual neuroscience, focusing on interpretability. Both biological and artificial neural systems are complex networks that exhibit intricate representations, and Binxu’s work is motivated by the belief that both can be understood through shared techniques. To achieve this, Binxu uses interpretability tools such as feature visualization to study the primate visual cortex, as well as geometric and circuit analysis tools to understand deep learning models such as generative adversarial networks (GANs) and diffusion models. The ultimate goal of this research is to gain a deeper understanding of intelligence in both systems and explore the shared principles between them.
About the Kempner Institute
The Kempner Institute seeks to better understand the basis of intelligence in natural and artificial systems by recruiting and training future generations of researchers to study intelligence from biological, cognitive, engineering, and computational perspectives. Its bold premise is that the fields of natural and artificial intelligence are intimately interconnected; the next generation of artificial intelligence (AI) will require the same principles that our brains use for fast, flexible natural reasoning, and understanding how our brains compute and reason can be elucidated by theories developed for AI. Join the Kempner mailing list to learn more, and to receive updates and news.
PRESS CONTACT:
Deborah Apsel Lang | (617) 495-7993 | deborah_apsel_lang@harvard.edu